A fundamental idea in the discussion of variability is the concept of deviation. Simply put, a deviation measure tells us how far away a given value is from the mean of the distribution—that is, .
To find the deviation of a set of values, we define the variance as the sum of the squared deviations and normalize it by dividing it by the size of the dataset. This is referred to as the variance. We need to use the sum of the squared deviations. By taking the sum of the deviations around the mean results in 0, since the negative and positive deviations cancel each other out. The sum of the squared deviations is defined as follows:
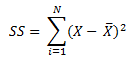
The preceding expression is equivalent to the following:

Formally, the variance is defined as follows:
- For sample variance, use the following formula:
- For population variance, use the following formula:

The reason why the denominator is N-1 for the sample variance instead of N is that, for sample variance, we want to use an unbiased estimator. For more details on this, take a look at http://en.wikipedia.org/wiki/Bias_of_an_estimator.
The values of this measure are in squared units. This emphasizes the fact that what we have calculated as the variance is the squared deviation. Therefore, to obtain the deviation in the same units as the original points of the dataset, we must take the square root, and this gives us what we call the standard deviation. Thus, the standard deviation of a sample is given by using the following formula:

However, for a population, the standard deviation is given by the following formula:
